The spatial/angular resolution of traditional optical imaging systems is limited by the Abbe-Rayleigh diffraction limit, and researchers generally seek to increase the numerical aperture or effective aperture of the imaging system to obtain higher resolution images. However, considering the cost and difficulty, this method becomes more and more challenging. The development of super-resolution (SR) imaging technology provides the possibility to surpass the diffraction limit and pave a new way to further increase the spatial/angular resolution of imaging systems.
For their contribution to super-resolution imaging, E. Betzig and S. W. Hell won the 2014 Nobel Prize in Chemistry.
Recently, the team of Prof. Jinping He from Nanjing Institute of Astronomical Optics & Technology, Chinese Academy of Sciences made a new progress on sub-diffraction-limit imaging via an external aperture modulation subsystem (EAMS) and related deep learning strategy. The researchers use the image plane of the optical imaging system as the input to the EAMS, with no need to change the original system structure, and the low resolution (LR) to high resolution (HR) image pairs required for training can be quickly acquired by adjusting a motorized iris diaphragm.
With the well-trained deep learning network, termed dpcCARTs-Net in this work, the HR image is used as the input to inference a super-resolution image beyond the diffraction limit. The related works were published in the Optics Express journal (Vol. 29, issue 20, PP 31099-31114, 2021)and applied for a national invention patent. So far, the team has proposed three new super-resolution imaging methods based on aperture modulation: "aperture modulation + intensity extrapolation" (Sci. Rep. 8, 15216, 2018), "aperture modulation + image iterative reconstruction" (J. opt. 23, 015701, 2021) and this method "aperture modulation + deep learning".
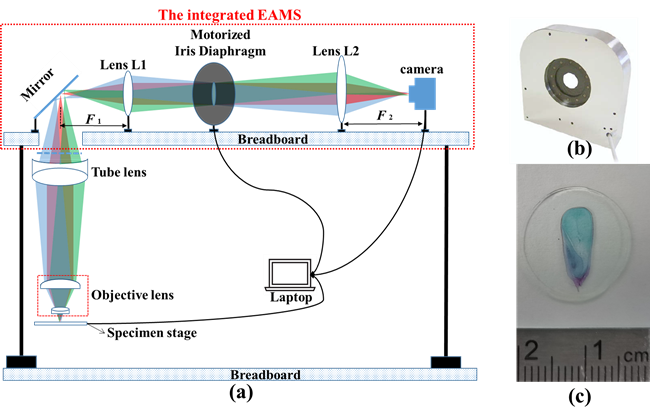
Fig. 1. The experimental setups. (a) Schematics of the imaging system and the EAMS. (b) The motorized iris diaphragms. (c) The longitudinal sections of maize seed used in our experiments. A ruler is put aside as a reference.
As introduced by Dr. Zhiqinag Wang, first author of the paper, this method doesn’t change the design of the original imaging system, and it only needs add a variable aperture or aperture modulation subsystem to realize the aperture modulation process. Thus, this framework can be implemented as an add-on module to existing or intending imaging systems with compact setup and low cost. In the training phase, the resolution enhancement ability and image fidelity of the deep learning network can be further improved by adding one "label data", which is convenient to be achieved by the EAMS proposed in this work.
Compared to iterative optimization SR algorithm, the SR method based on deep learning can provide well-trained non-iterative reconstruction tools to perform fast resolution enhancement, without estimating point spread function (PSF) or numerical modeling of imaging process.
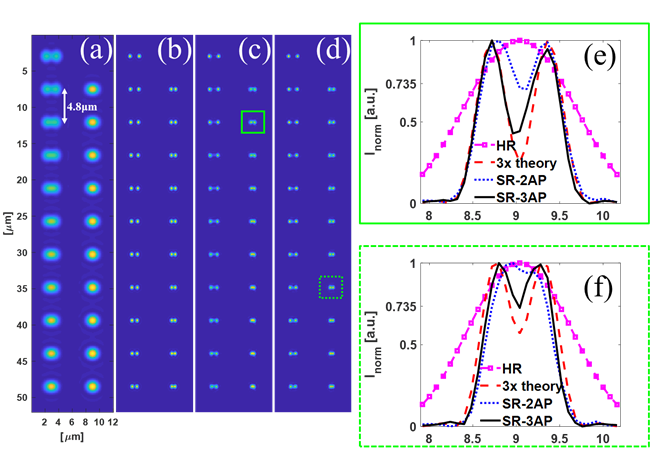
Fig. 2. The results of the well-trained dpcCARTs-Net on the two-point source pairs. The interpolated results of (a) the diffraction-limited HR input, (b) the 3 times theoretically super-resolved image. The extrapolated SR output of the dpcCARTs-net trained with (c) 2-aperture modulation strategy and (d) 3-aperture modulation strategy. The cross-sectional profiles of the resolution enhancement of (e) 2.2 times and (f) 2.7 times. Here 0.735 is the normalized intensity of the saddle-point according to Rayleigh criterion. (a)-(d) are pseudo-color images and normalized to 1. These two-point source pairs are equally arranged in longitudinal direction with a separation distance of 4.8 μm.
To test and verify the SR imaging ability of this method on complex targets, the team further carried out experimental researches on biological specimens by cooperating with Nanjing Forestry University and Shanghai Jiaotong University. Fig. 3 shows the imaging results of the longitudinal section of maize seed. Compared with the diffraction limited HR input, the extrapolated SR output of dpcCARTs-Net net is significantly improved in resolution and contrast.
The SR performance of dpcCARTs-Net net trained with 3-aperture modulation strategy is also better than that of 2-aperture modulation strategy, and the resolution enhancement ability is approaching 1.5 times. The extrapolated SR image can provide clearer biological structure information, which is helpful to further study the biological information of specimens, such as the evaluation of starch content.
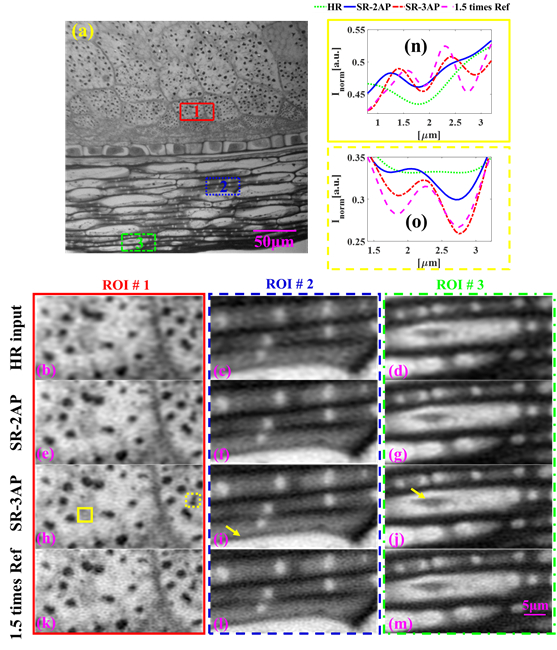
Fig. 3. The results of dpcCARTs-net on a new longitudinal section of maize seed. (a) An example extrapolated SR output image of the dpcCARTs-net trained with 3-aperture modulation strategy. Comparison of zoom-in ROIs: (b, c, d) the diffraction-limited HR input. The extrapolated SR output of the dpcCARTs-net trained with (e, f, g) 2-aperture modulation strategy and (h, i, j) 3-aperture modulation strategy. The cross-sectional profile of the two (n) white and (o) black feature spots, with the same box as marked in (h). The yellow arrows in (i, j) point to some blurred gaps and shapes that are brought clearer by the well trained dpcCARTs-net. All images are normalized to 1.
In the future, the team will focus on applying relevant methods to large field of view astronomical imaging, which is expected to realize ultra-high resolution astronomical imaging at a much lower cost. These works will provide a foundational reference for the design of intelligent astronomical instruments and related research of smart astronomical data processing algorithm in the future.
The work is supported by National Natural Science Foundation of China (11903062, 11773045, 11933005).